the Creative Commons Attribution 4.0 License.
the Creative Commons Attribution 4.0 License.
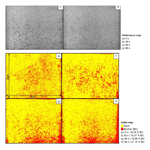
Testing of photogrammetry for differentiation of soil organic carbon and biochar in sandy substrates
Wolfgang Fister
Nina Goldman
Marius Mayer
Manuel Suter
Nikolaus J. Kuhn
Landforms in different environmental ecosystems are formed through selective erosion and transport mechanisms by wind and water. To understand their development, biochemical composition, and effect on neighbouring ecosystems, it is essential to better understand the forming processes and to be able to monitor the behaviour and transport of particles through the landscape. Conventional methods to track and differentiate between organic and inorganic particles usually involve extensive sampling campaigns that disturb the processes themselves. Therefore, this study aimed to prove that the method of photogrammetry, which is non-contact and non-invasive, is able to quantify erosion rates and can also be used to differentiate between organic and inorganic material. To prove this concept, a bottom-up experimental rainfall simulation set-up was conducted with sieved sand substrates of very narrow particle size distribution and biochar under highly controlled conditions. By using two-dimensional index and reflectance maps of the surface computed with the software Pix4D, the erosion of biochar was estimated and compared to the actual erosion amount that was transported along the plot. The results show that photogrammetry has the potential to distinguish between a sandy substrate and biochar. An approximate decrease of 5 % in biochar on the plot surfaces was detected in three out of four rainfall experiments within the first 30 s. After these 30 s, the quality of detection was significantly reduced due to shadow effects, which were falsely classified as biochar as well. For future investigations on this topic, it will be necessary to improve the lighting of the plot and the post-processing of the images to reduce the error caused by shadows. Additional approaches that should be taken into account for further test experiments are the full exclusion of external light, which means working in the dark, and the use of hyperspectral cameras to detect different carbon types.
- Article
(5906 KB) - Full-text XML
- BibTeX
- EndNote
The selective transport of soil particles is an important process for the development of many different landforms and their biogeochemistry in varying ecosystems that are affected by wind or water (Kirkels et al., 2014; Shi et al., 2012). These landforms may, for example, range from lowland agricultural fields (Estrany et al., 2010), over drylands (Sterk et al., 2001) and coastal areas (Williams et al., 2018), up to braided river systems and bare glacier forefields in high-mountain or arctic environments (Bernasconi and BigLink Project Members, 2008). In order to understand the development of these landforms it is essential to better understand the forming processes (Schwanghart et al., 2013) and to be able to track and monitor the behaviour and transport of particles through the landscape. Moving particles not only consist of inorganic components, but also of organic material such as litter or charcoal (black carbon), which becomes more and more important against the backdrop of global climate change and global biochemical cycles (Cornelissen et al., 2005; Forbes et al., 2006; Lehmann et al., 2008). However, due to their different properties, e.g. density and shape, the basic transport processes of organic matter are currently not well understood (Hu and Kuhn, 2014). To enable well-based quantitative ascertainment and modelling on sediment, soil organic carbon or black carbon transport rates, further monitoring and experiments are needed (Foereid et al., 2011). This research requires methods that facilitate the differentiation between sediment and varying types or fractions of organic carbon.
The method most often used to detect and quantify soil organic carbon is combusting the sediment. This can be done by either quantifying the net weight loss before and after combustion, e.g. in a muffle furnace (e.g. Cargua Catagña et al., 2017), or by back-calculating the inorganic and organic carbon content by measuring CO2 emissions at different temperature grades in combustion analysers (e.g. Hoffmann et al., 2014; Quijano et al., 2016). Both of these methods have been successfully used for many years. However, their major drawback is that the sample itself is destroyed during the analysis and no further analyses are possible. In some situations this issue can be overcome by taking more representative subsamples, but if the space for taking samples or the amount of sediment is limited, this might not be a valid option. Sample extraction from a location is always an invasive process, which might be problematic for further experimental investigations. This means that the surface structure (e.g. crust) of an eroding site is disturbed, or in the worst case destroyed, and the ongoing processes are altered and thus compromised. Often it is not possible to take samples from the soil surface during running experiments and the valuable development of the processes and their effects on the soil over time cannot be monitored. Another current approach deals with the method of thermogravimetry. It determines material weight loss as a function of temperature in a controlled environment (Leifeld, 2007). By measuring the weight loss at certain combustion temperatures, different types of soil organic matter can be distinguished. Zimmermann et al. (2007) use a mixed-methods approach, which combines both physical and chemical fractionation methods and is considered the “best practice” approach (Stockmann et al., 2013) for fractioning soil organic carbon. This fractionation becomes very important when looking at carbon sequestration and varying retention times of carbon in the soil, especially as carbon differs in stability and reactivity, which might influence its behaviour in soil erosion and transport processes.
A substance that has recently been looked at more closely with regards to the mentioned research questions is biochar (BC). BC is a carbonaceous material which is produced through the heating of biomass with little or no presence of air (low oxygen (O2)-supply) to temperatures of 300–700 ∘C (Boateng et al., 2015). BC is chemically and environmentally highly stable, because of its polyaromatic structure (Simpson and Hatcher, 2004) and could perhaps be used to reduce the CO2 problem by subsurface storage of C (Lehmann, 2007; Preston and Schmidt, 2006; Seifritz, 1993; Sohi et al., 2010). In order to act as a soil amendment and C-storage option, BC needs to stay on the field and should not be eroded and transported to neighbouring trenches or water courses (Fister et al., 2013a). Rumpel et al. (2015) showed that BC erosion has great influence on the C budget of BC-amended soils on one hand and on depositional sediments or soils on the other hand.
Rainfall simulation experiments or field measurements are a good option for determining the reduction in BC efficacy, because of their controlled conditions and repeatability of the experiments (Kuhn et al., 2014). However, a fundamental requirement for this kind of research is the availability of a method that is able to differentiate between BC and regular soil organic carbon (SOC) (Fister et al., 2013a; Rumpel et al., 2006).
Preliminary tests in our laboratory showed that none of the combustion methods mentioned above were able to satisfactorily differentiate between the two carbon types. The applied BC contents (from the company Swiss Biochar) in the experiments were too low (0.1–2 wt. % BC) to show distinctive differences in the measurements. The above-mentioned mixed-methods approach by Zimmermann et al. (2007) would most probably be able to detect these differences. However, the method is laborious and time consuming, which makes it less useful when dealing with large sample numbers. These difficulties call for the development of new methods, which ideally would fulfil all the necessary requirements, like non-contact, non-invasive preservation of the sediment sample, and differentiation between soil organic carbon, biochar, and soil substrates at low concentrations. Therefore, the aim of this initial pilot study, carried out in the experimental rainfall laboratory at the University of Basel, was to evaluate whether the method of photogrammetry could be used to quantify and differentiate between erosion of biochar and a sandy substrate.
A bottom-up experimental rainfall simulation set-up was chosen to conceptually verify whether photogrammetry could be used for this purpose. The complexity of the processes was reduced by using sieved sand substrates of narrow particle size distribution under highly controlled, simulated rainfall and surface flow conditions in the laboratory. Using more complex soil substrates and field experiments was avoided, because the high variability of the soils and the lack of control would have made it very difficult to test the basic concept. This decision was supported by some pretests in our laboratory with a Leica DMS 1000 microscope under ideal lighting conditions with different mixtures of quartz sand, soil, and biochar. The results (Table 1) indicate that the detection quality of biochar decreases more rapidly with more heterogenic and finer particles. Coarse BC can be registered accurately with the semi-automated (manual threshold definition) and automated (computerized threshold definition) detection algorithms using the software ImageJ version 1.8.0. The observed error to the manual reference measurement, using the Leica Application Suite version 4.4, was 3.8 % for semi-automatic and 7.8 % for the automated detection. The semi-automated detection also worked for quartz sand with mixed BC, but produced errors up to 39 % for soil mixtures. The automated threshold definition proved unreliable for mixed BC and both soil mixtures.
Table 1Results of photogrammetric pretests that differentiate between quartz sand (D50350 µm), soil (D5065 µm), and coarse (range from 500 to 1500 µm) as well as mixed (range from 0 to 1500 µm) biochar under optimal lighting conditions using a Leica DMS 1000 microscope.

1 Using Leica Application Suite software version 4.4. 2 Using ImageJ software version 1.8.0.
2.1 Photogrammetry
Using photogrammetry is nothing new to soil erosion research, but it usually concentrates on larger features such as gullies, rills, and badland erosion, dealing with larger topographical changes. Hänsel et al. (2016) and Prosdocimi et al. (2017), however, used this method on small-scale flume experiments and created digital elevation models to try to quantify soil erosion rates. In general, results from photogrammetric methods still seem to be quite variable and therefore lack a certain reliability. One important reason for this variability is that particles are not only transported on the soil surface, but they can also be buried and remobilized, or they can even be trapped for the whole erosion event. In addition, digital elevation models only provide information on the total erosion amount by changing the topography, but do not facilitate the differentiation between different eroded material, for example biochar or soil organic carbon and the substrate. Therefore, this study takes a slightly different multi-temporal approach by using reflectance maps. By using reflectance maps, it is expected to be able to differentiate between the different types of particles. The use of photogrammetry also solves the above-mentioned issues, because it is non-invasive, which means that it could theoretically be used to monitor erosion processes and thus landform development over longer periods of time without disrupting ongoing processes and without the need for personnel to take samples on site.
To create an adequate representation of the flume surface in this experiment, nine photos were taken on a grid of three by three. A rainfall experiment lasted for 240 s and the images were taken before the experiment started and at the intervals 30, 60, 90, 120, 150, 180, and 240 s into the experiment. The camera height was set to 26 cm from lens to substrate surface. This was the shortest distance that yielded sharp images. The photos were taken with a GoPro Hero4 Silver camera, which was set to medium field of view (127∘) and 7 megapixels. Like in the study by Prosdocimi et al. (2017) inhomogeneous lighting was a great challenge. To counteract this problem, two adjustments were made to the set-up. Firstly, a tarpaulin was set up behind the erosion flume to reduce direct lighting and secondly, an annular orifice was mounted directly underneath the camera (Fig. 1). It was assumed that these methods would reduce shadows on the plot to enhance picture quality.
The pictures were loaded into the photogrammetry software Pix4Dmapper. The images were not georeferenced, as the flume dimensions are too small to accurately use GPS points. GPS points are only accurate to 0.02 m, which was not accurate enough for the small surface area of 0.2 m2. However, it is advisable to georeference plots in large-scale experiments to provide scale and orientation as well as reduce error accumulation with ground control points (Pix4D, 2017).
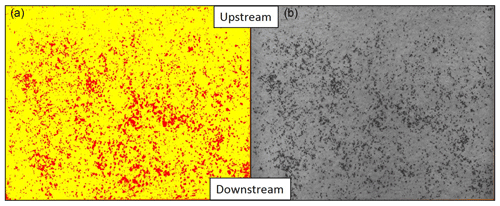
Figure 2Example of an index map (a) and a reflectance map (b). The index map classifies 14.3 % of the marked area as biochar (red), and 85.7 % of the area as sand (yellow).
After uploading all the photos into the Pix4D programme, it generates a reflectance map (Fig. 2), which is a 2-D map that describes the reflectance of the area to be mapped. Here, compared to the orthomosaic, no colour balancing is applied as the value of each pixel faithfully represents the reflectance from the object. The aim of the reflectance map is to assess a particular feature based on the pixel value in the images, which is influenced by some factors such as aperture or ISO. The Pix4Dmapper therefore uses a model to account for these factors and to produce an accurate reflectance map. This provides the basis for an index map, wherein the value of every pixel on the map is obtained from the associated reflectance map. The number of classes in the index map needed to be adjusted (from default of 5 to 2), as the erosion substrate contained only two different materials (BC and sand), which have different reflectance values (Pix4D, 2017). The method of classification also needs to be changed to a natural-breaks optimization method, also known as Jenks, which clusters the data to minimize the variance within a class and maximizing the differences between classes (Jenks, 1967). In addition, the minimum and maximum of the index range were adjusted to ignore values outside this range. Adapting this range to a new minimum of 30 and a new maximum of 135 yielded a more accurate result regarding the surface coverage of BC and sand. This index map gives us a percentage value, which shows how much of the flume's surface is BC and how much is sand based on the different reflective values of BC and sand.
2.2 Characterization of test substrate
The biochar used in this study was from Swiss Biochar GmbH and is made from wood and protein-rich stone fruits. The BC was pyrolysed at 450–600 ∘C and had a bulk density of 0.281 g cm−3. The density is comparable to other measured BC bulk density values, which can range from 0.09 up to 0.5 g cm−3 (Chia et al., 2015). The particle sizes were measured using a freefall dry feeder module (Scirocco 2000) of the Malvern Mastersizer 2000 optical bench. Particles above 2 mm cannot be measured with the wet module (Hydro 2000 G) since they clog up the measuring device. The BC was sieved to the fraction of 1–2 mm and showed a D50-value of 1.22 mm.
Quartz sand was used as a substrate to receive the best possible contrast between the particles under investigation in this pilot study. Apart from this more practical reason of having a good contrast, sandy soils would also be the ones that could benefit most from biochar application. Therefore, the choice of very narrowly sized sand substrates was more representative than using silt or clay sized substrates for a start. The sand was fire dried and naturally rounded. The SiO2 content was 97 % and it was sieved to a D50 of 0.673 mm. The mean values of five replicate measurements showed a bulk density of 1.51 g cm−3.
2.3 Rainfall experiments
A high-precision drip-type rainfall simulator (Fig. 3) was used on a microscale flume with controllable surface flow (Fig. 4) to be able to precisely control and monitor the thin surface flows and rainfall characteristics. For the experiments, deionized water was used to minimize clogging the needles with lime. Since the test material only consisted of BC and sand, the high sodium content did not matter with regards to the dispersion of particles. The fall height of the raindrops for all experiments was 6 m. According to van Boxel (1998) this would mean that the raindrops, with an average drop diameter of 2.6 mm, would reach 90 % to 95 % of their terminal velocity. The soil substrate was positioned in the 0.4 m long test section near the end of the flume. The configuration was adapted from a design originally used by Moss and Green (1983) and further adapted and refined by Kinnell (1988). The development of this simulator did not try to fully imitate natural rainfall, but aims to produce the highest possible reproducibility and precise drop formation and is, therefore, well suited for process studies. Since the focus of this paper is on the analyses of photogrammetry, please refer to Greenwood et al. (2013) and Fister et al. (2013b) for further details on the rainfall simulator.
2.4 Sampling of eroded material and separation of biochar
All of the eroded sand particles and most of the BC were caught in the sediment collection chamber directly behind the erosion flume. In order to avoid loss of biochar through the outlet, a fine sieve was placed underneath the discharge water. After each experimental run, the material from the sediment chamber was siphoned out into small containers for separation of particles and weighting. Material loss by splash was not collected due to its very limited amount in relation to the flow-driven discharge.
In order to quantify the amount of eroded biochar in the sediment, it needed to be separated from the sand. The first method used was dry sieving. This was only possible for the fine-sand mixtures, because the large sand particles would have been retained on the 630 µm sieve together with the 1–2 mm BC particles. The larger particles were separated using an inclined slope with flowing water. Based on the model of simple sluice boxes for washing gold, a 170 cm by 5 cm by 4 cm sluice made from a metal U-profile was used. Five removable metal block inlets with heights of 5 mm were placed inside the profile at equal intervals. One end of the U-profile was elevated to create a gentle slope and a hose, connected to a tap, was attached to the upstream end. Then the material was added and an instant discharge of the lighter BC particles was noticeable, while the heavier sand deposited and accumulated behind the metal barriers (Fig. 5). This method turned out to separate the materials most thoroughly without any loss of material.
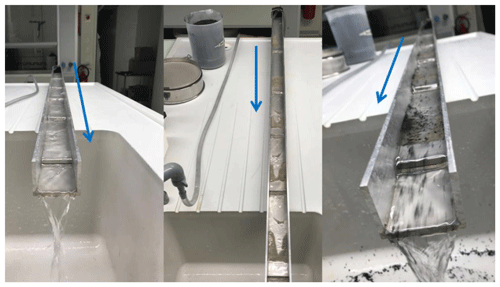
Figure 5Sluice set-up, which separates BC from sand where the sand is trapped behind the step and the BC is suspended in the flow and carried downstream. The BC is then caught in the sieve at the end of the sluice and placed in a drying chamber at 40 ∘C.
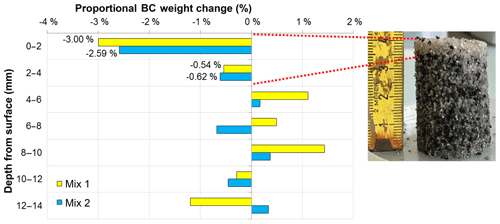
Figure 6Average values of five profile samples that were taken from the erosion plot after 240 s of rainfall at 100 mm h−1. Two different BC concentrations (mix 1 and 2) were tested. These profile samples were sliced into 2 mm layers. The BC and sand in these layers were then separated by combustion in a muffle furnace. Thereby we could determine the vertical depletion of our erosion flume.
3.1 Quantification of BC by photogrammetry
In order to assess the accuracy of the photogrammetry method, the BC coverage from Pix4D was compared to the actual BC erosion amounts. We are aware that they cannot be identical as the photogrammetry method only captures the surface area, whereas the BC collected at the flume outlet is also derived from the subsurface area, as mentioned in the introduction chapter. Nevertheless, the comparison gives a good indication and allows the quality of the photogrammetry method to be assessed. Initial tests showed that, within the 240 s, most of the depletion occurs within the top 2 mm from the surface and only very little in the depth between 2 and 4 mm (Fig. 6). Wang et al. (2013) observed a depletion of the top 15 mm of the surface after 4 h of rainfall. This difference in depletion depth highlights the importance of knowing the rate of depletion for a given experimental set-up. Knowing the rate of depletion in the subsurface allows for a more accurate calculation of how much BC has been eroded, based on the photogrammetry method.
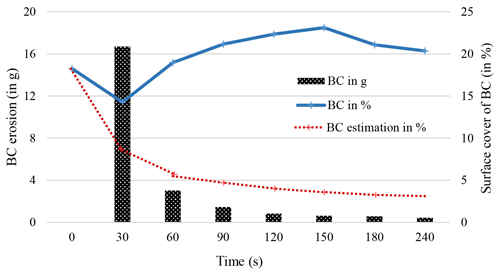
Figure 7Exemplary illustration of actual surface coverage as calculated by Pix4D (blue line) and estimated surface coverage of BC (dotted red line) with measured BC discharge rates (in grams) across the time series.
In Fig. 7 the photogrammetry results are compared to the actual BC erosion for one exemplary rainfall experiment. It shows the percentage of BC coverage calculated by Pix4D (blue line) versus the estimated BC coverage (red line). The black columns indicate the amount of BC erosion in grams. This shows that, after an initial flush containing more than 16 g of BC, the detachment of BC decreases are less pronounced over time. This erosion pattern over time is to be expected on a boundary-limited flume, since BC cannot be replenished from upstream areas, as would be the case on field sites. This erosion pattern can also be observed in the other rainfall experiments with the same substrate composition (Fig. 8) and is in agreement with the literature (e.g. Hu et al., 2013). The actual depletion of the surface coverage in BC was calculated from the amount of erosion by the surface area and is indicated by the red line. The surface coverage of BC, which was calculated using the index map (blue line), also shows an initial decrease from 18.25 % at 0 s to 14.27 % at 30 s. After the 30 s measurement interval, values increase to a maximum of 23.11 % at 150 s, which is almost 5 % higher than the BC coverage at the start of the experiment. The lower erosion rate in the first 30 s is most probably caused by the fact that photogrammetry only captures the BC depletion on the surface and not the subsurface depletion in the top 2 mm or even 4 mm from the surface (Fig. 6). The observed increase in BC coverage of about 5 % cannot be explained based on the erosion process itself.
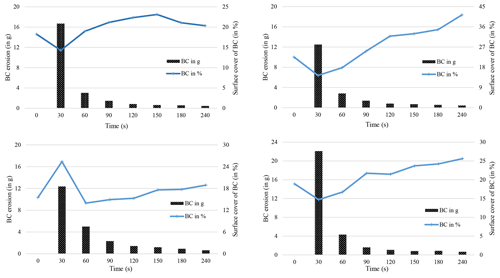
Figure 8Results from the photogrammetry analysis which show the amount of BC on the surface (in %) combined with BC erosion (in grams) for one set of runs (R46 a, R47 b, R48 c, R49 d).
Figure 8 shows four repetitions of the same rainfall experiment, i.e. with the same BC and sand particle size, highlighting that this behaviour is not a single artefact. Most of the runs show an initial decrease in surface coverage of BC (blue line) for the first interval (average of 5.44 %), but an increase afterwards. Run 48 (bottom left) is an exception to this pattern and shows a severe increase of 9.88 % in BC coverage in the first interval before a similarly strong decrease occurs in the second measurement interval. This large increase in BC coverage is most likely a cause of increased exposure of subsurface BC due to high erosion of sand particles. The reflectance map shows clear evidence of higher BC surface coverage after 30 s. An additional indication for this theory is the relatively low BC erosion amount for run 48 (12.37 g). For the other runs the initial flush of BC after 30 s resulted in higher discharge amounts of 16.69 g for run 46, 12.49 g for run 47, and 22.10 g for run 49.
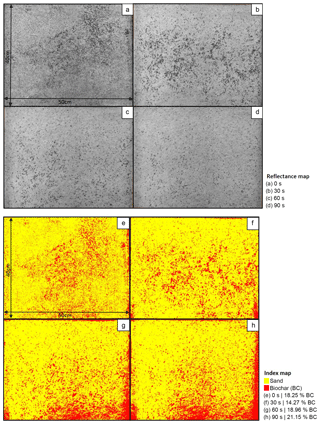
Figure 9Reflectance maps (a–d) and index maps (e–h) of the run presented in Fig. 7. Surface coverage of BC is in red and sand is in yellow across the time series. The legend shows the time and the BC coverage of the index map in %.
In order to see if an actual BC increase on the surface occurred during the experiment or if some other reason caused the increase in BC coverage on the surface, we compared the reflectance and the index maps for the run from Fig. 7 with each other. The comparison of the flume surface before the rainfall and after the first three intervals of 30, 60, and 90 s is shown in Fig. 9. The reflectance maps clearly show that the BC coverage (black dots) decreases with increasing rainfall duration. In contrast, the BC coverage in the index maps (red dots) increases over time from 18.25 % before the rainfall to 21.15 % coverage after 90 s. This confirms that the Pix4D software overestimates the presence of BC on the surface. Most likely, shadowy sand particles were misclassified as BC particles. This misclassification occurred mostly along the flume's boundaries. Even the additional use of a tarpaulin to reduce direct light on the plot and the use of the annular orifice (Fig. 1) to light up the flume's surface did not sufficiently reduce the shadow effect near the flume boundaries.
3.2 Usability of photogrammetry for BC and SOC monitoring
The experiences obtained in this pilot laboratory study show that using photogrammetry to analyse images with reflectance and index maps is relatively easy and does not require expensive professional equipment. The GoPro cameras can be equipped with a water-resistant casing, which is advisable when obtaining data in a rain laboratory or outdoors to monitor SOC movement. Mounting the GoPro cases on metal frames with hook-and-loop tape, for example, makes it easy to install and uninstall the camera for usage. The external control of the camera via an application ensures that the camera is not moved during the experiment and ongoing processes are not disturbed. The live preview of the images being taken acts as an extra control and ensures that the pictures depict the flume's test section.
The handling of the image analysis software Pix4D can be either learned through tutorials or with the support website. However, it is expensive, especially if multiple licences are needed. The processing power and storage capacity required from the work station is very high, so that processes are severely slowed down when used on a regular laptop, especially as the identification of key points and subsequent merging of the images requires a powerful processor.
Using the photogrammetry software Pix4D to assess the amount of BC loss due to rainfall events showed promise, but not the most accurate results. The software was able to reproduce the decrease in BC surface coverage in the first measurement interval and the deviations can be partly explained by the observed processes (see above). However, for the later intervals with less significant changes in BC surface cover, the software seems to overestimate the real BC coverage due to shadow effects, despite the efforts to facilitate homogenous lighting on the plot surface with a tarpaulin and an annular orifice. For future research it will be fundamental to reduce the shadows on the plot and to improve the detection of BC particles. Therefore, we suggest creating a more elaborate lighting set-up that prevents shadows, especially near the plot boundaries, on one hand, and reduces the sensitivity of the classification tool so it can better distinguish between shadowy sand and BC, on the other hand. Instead of trying to calibrate and improve Pix4D, an alternative could be to use a different software, which is more successful in this respect. In the long run, there are many other options that should be tested to evaluate their potential to increase the detection quality of BC. For example, the effect of using optical or software filters to reduce the shadows in the captured images. Another option could be to conduct experiments in the dark, which would enable full control over lighting conditions. Such an approach was successfully tested with fluorescent tracers in small-scale plot experiments (Hardy et al., 2017) as well as in large field experiments on soil erosion (Hardy et al., 2019). Another very promising approach comes from the field of soil spectroscopy. Using hyperspectral images could be of special interest when using more complex substrates with soil components and SOC, which are more difficult to differentiate, because of their lower contrasts. Hobley et al. (2018), for example, used hyperspectral images with a variety of machine-learning approaches to predict organic carbon distribution in soil cores, and Burud et al. (2016) were able to map biochar qualitatively and quantitatively in a soil monolith. With the development of simple, lightweight, and portable spectro-goniometers (Anderson et al., 2012) it seems very likely that these devices could also be used for soil erosion plot measurements. In order to improve the reliability of experiments on photogrammetry and soil erosion, it will also be necessary to solve the issue of burial and remobilization of particles during the event. An option for future small-scale laboratory experiments could be using the above-mentioned method to take profile samples before and after experiments and to analyse them on their BC contents. Assuming that the vertical concentration change is similar over the whole surface, which is only possible on small-scale plots with homogenous conditions, a correction factor for the total BC loss could be estimated.
The aim of this pilot study was to evaluate whether the method of photogrammetry can be used to quantify and differentiate between erosion of biochar and a sandy substrate. This would be the first step to prove that soil organic carbon can be monitored in the landscape via photogrammetry. The results show that it was possible to quantify BC coverage based on index maps for the first 30 s interval. Additionally, the preferential erosion of BC could be observed and quantified during the experiments. However, during further time steps in the rainfall experiments, the BC coverages showed an increase and thus deviated quite significantly from the expected values, which were obtained using the measured BC erosion. The overestimation of BC coverage is most probably caused because of the inclusion of shadows by the Pix4D algorithm. The installation of a tarpaulin as protection against the influence of direct light and the use of an annular orifice did not work satisfactorily. Therefore, it seems to be very challenging to gain more accurate results with the present set-up.
In conclusion, photogrammetry could become a very important method in landscape monitoring of soil organic carbon, related erosion, and transport processes, especially because it fulfils all the other important requirements, for instance non-contact, non-invasive preservation of the sediment sample, and differentiation between soil organic carbon at low concentrations. With an appropriate set-up it seems very likely that it could, for example, be possible to monitor litter movement or composition through longer time periods and from greater distances (metres). To go even further, with present advancements in image particle tracking, it could also be possible to track individual particles and calculate their transport rates and distances travelled. However, this requires more laboratory and field experiments to test and improve the methodology. Advancements in optical and software filter techniques, as well as calibration of the Pix4D software or the use of alternative programs, need to be evaluated on their feasibility. Instead of trying to improve the lighting conditions on the plot by using curtains or special illumination, we think it would be more advisable to pursue the experiments in the dark, to have full control over lighting conditions. Another promising option for improving the detection quality of BC and SOC in soil substrates under laboratory and field conditions seems to be the use of hyperspectral cameras.
Data analysis of biochar contents is based on image analysis with Pix4D. All results from image analysis and profile depth measurements are presented in the article. The original images for our own analysis can be requested from the corresponding author.
NJK instigated the initial studies on biochar and provided the experimental research facilities. NG and MM performed the rainfall experiments and data analysis under supervision of WF for their individual theses. WF developed the specific research question for the article and wrote the article with support from NG, who also produced all figures and performed the Pix4D analysis. MS provided additional data and experiments to back up the results. NJK improved and commented on the experiments and manuscript throughout the process.
The authors declare that they have no conflict of interest.
The authors would like to thank Jens Leifeld from Agroscope in
Zürich for his support with the initial thermogravimetry measurements
and Ruth Strunk, Brigitte Kuhn, and Hans-Rudolf Rüegg from
the University of Basel for their technical support in the laboratory and
with the Pix4D image analysis.
Edited by: Christoph Graf
Reviewed by: two anonymous referees
Anderson, K., Croft, H., Milton, E. J., and Kuhn, N. J.: A simple spectro-goniometer for collection of multiple view angel reflectance factors, Remote Sens. Lett., 3, 131–140, 2012.
Bernasconi, S. M. and BigLink Project Members: Weathering, soil formation and initial ecosystem evolution on a glacier forefield: a case study from the Damma Glacier, Switzerland, Mineral. Mag., 72, 19–22, 2008.
Boateng, A. A., Garcia-Perez, M., Masek, O., Brown, R., and del Campo, B.: Biochar production technology, in: Biochar for environmental management. Science, technology and implementation, edited by: Lehmann, J. and Joseph, S., 2nd Edition, Routledge, 63–87, 2015.
Burud, I., Moni, C., Flo, A., Futsaether, C., and Steffens, M.: Qualitative and quantitative mapping of biochar in a soil profile using hyperspectral imaging, Soil Till. Res., 155, 523–531, 2016.
Cargua Catagña, F. E., Rodríguez Llerena, M. V., Damián Carrión, D. A., Recalde Moreno, C. G., and Santillán Lima, G. P.: Analytical methods comparison for soil organic carbon determination in Andean Forest of Sangay National Park-Ecuador, Acta Agron., 66, 408–413, 2017.
Chia, C. H., Downie, A., and Munroe, P.: Characteristics of biochar: physical and structural properties, in: Biochar for environmental management, Science, technology and implementation, edited by: Lehmann, J. and Joseph, S., 2nd Edition, Routledge, 89–109, 2015.
Cornelissen, G., Gustafsson, A., Bucheli, T., Jonker, M. T. O., Koelmans, A. A., and van Noort, P. C. M.: Extensive Sorption of Organic Compounds to Black Carbon, Coal, and Kerogen in Sediments and Soils: Mechanisms and Consequences for Distribution, Bioaccumulation, and Biodegradation, Environ. Sci. Technol., 39, 6881–6895, 2005.
Estrany, J., Garcia, C., and Walling, D. E.: An investigation of soil erosion and redistribution in a Mediterranean lowland agricultural catchment using caesium-137, Internat. J. Sed. Res., 25, 1–16, 2010.
Fister, W., Heckrath, G., Greenwood, P., and Kuhn, N. J.: Erodibility of biochar from a sandy soil in Denmark. In: United Nations Convention to Combat Desertification: Extended abstracts of UNCCD 2nd Scientific Conference, UNCCD, 249–253, 2013a.
Fister, W., Rüegg, H., Tresch, S., and Greenwood, P.: Eine Präzisionsanlage zur experimentellen Untersuchung von Bodenerosionsprozessen, Regio Basiliensis, 54, 19–26, 2013b (in German).
Forbes, M. S., Raison, R. J., and Skjemstad, J. O.: Formation, transformation and transport of black carbon (charcoal) in terrestrial and aquatic ecosystems, Sci. Total Environ., 370, 190–206, 2006.
Foereid, B., Lehmann, J., and Major, J.: Modeling black carbon degradation and movement in soil, Plant Soil, 345, 223–236, 2011.
Greenwood, P., Fister, W., Kinnell, P. I. A., Rüegg, H.-R., and Kuhn, N. J.: Developing and testing a precision erosion measurement facility for elucidating mobilization mechanisms in shallow-flow conditions, in: Proceedings of the 4th Conference on Desertification and Land Degradation, 19 June 2012, Ghent Belgium, edited by: Gabriels, D. and Vermang, J., 105–111, 2013.
Hänsel, P., Schindewolf, M., Eltner, A., Kaiser, A., and Schmidt, J.: Feasibility of high-resolution soil erosion measurements by means of rainfall simulations and SfM photogrammetry, Hydrology, 3, 1–16, 2016.
Hardy, R. A., James, M. R., Pates, J. M., and Quinton, J. N.: Using real time particle tracking to understand soil particle movements during rainfall events, Catena, 150, 32–38, 2017.
Hardy, R. A., Quinton, J. N., James, M. R., Fiener, P., and Pates, J. M.: High precision tracing of soil and sediment movement using fluorescent tracers at hillslope scale, Earth Surf. Proc. Land., https://doi.org/10.1002/esp.4557, 2019.
Hoffmann, U., Hoffmann, T., Jurasinski, G., Glatzel, S., and Kuhn, N. J.: Assessing the spatial variability of soil organic carbon stocks in an alpine setting (Grindelwald, Swiss Alps), Geoderma, 232–234, 270–283, 2014.
Hu, Y. and Kuhn, N. J.: Aggregates reduce transport distance of soil organic carbon: are our balances correct?, Biogeosciences, 11, 6209–6219, https://doi.org/10.5194/bg-11-6209-2014, 2014.
Hu, Y., Fister, W., and Kuhn, N. J.: Temporal variation of SOC enrichment from interrill erosion over prolonged rainfall simulations, Agriculture, 3, 726–740, 2013.
Jenks, G. F.: The Data Model Concept in Statistical Mapping, International Yearbook of Cartography, 7, 186–190, 1967.
Kinnell, P. I. A.: The influence of flow discharge on sediment concentrations in raindrop induced flow transport, Aust. J. Soil Res., 26, 575–582, 1988.
Kirkels, F., Cammeraat, L. H., and Kuhn, N. J.: The fate of soil organic carbon upon erosion, transport and deposition in agricultural landscapes. A review of different concepts, Geomorphology, 226, 94–105, 2014.
Kuhn, N. J., Greenwood, P., and Fister, W.: Use of Field Experiments in Soil Erosion Research, in: Geomorphological Fieldwork, Developments in Earth Surface Processes, 18, edited by: Thornbush, M. J, Allen, C. D., and Fitzpatrick, F. A., Elsevier, Amsterdam, 175–200, 2014.
Lehmann, J.: Bio-energy in the black, Front. Ecol. Environ., 5, 381–387, 2007.
Lehmann, J., Skjemstad, J., Sohi, S., Carter, J., Barson, M., Falloon, P., Coleman, K., Woodbury, P., and Krull, E.: Australian climate-carbon cycle feedback reduced by soil black carbon, Nat. Geosci., 1, 832–835, 2008.
Leifeld, J.: Thermal stability of black carbon characterized by oxidative differential scanning calorimetry, Organ. Geochem., 38, 112–127, 2007.
Moss, A. J. and Green, P.: Movement of solids in air and water by raindrop impact. Effects of drop-size and water-depth variations, Aust. J. Soil Res., 21, 257–269, 1983.
Pix4D: Support, available at: https://pix4d.com/support/, last access: 14 December 2017.
Preston, C. M. and Schmidt, M. W. I.: Black (pyrogenic) carbon: a synthesis of current knowledge and uncertainties with special consideration of boreal regions, Biogeosciences, 3, 397–420, https://doi.org/10.5194/bg-3-397-2006, 2006.
Prosdocimi, M., Burguet, M., Di Prima, S., Sofia, G., Terol, E., Comino, J. R., Cérda, A., and Tarolli, P.: Rainfall simulation and Structure-from-Motion photogrammetry for the analysis of soil water erosion in Mediterranean vineyards, Sci. Total Environ., 574, 204–215, 2017.
Quijano, L., Gaspar, L., and Navas, A.: Lateral and depth patterns of soil organic carbon fractions in a mountain Mediterranean agrosystem, J. Agric. Sci., 154, 287–304, 2016.
Rumpel, C., Chaplot, V., Planchon, O., Bernadou, J., Valentin, C., and Mariotti, A.: Preferential erosion of black carbon on steep slopes with slash and burn agriculture, Catena, 65, 30–40, 2006.
Rumpel, C., Ba, A., Darboux, F., Chaplot, V., and Planchon, O.: Movement of biochar in the environment, in: Biochar for environmental management, edited by: Lehmann J. and Joseph, S., Science, technology and implementation, Routledge, 283–299, 2015.
Schwanghart, W., Groom, G., Kuhn, N. J., and Heckrath, G.: Flow network derivation from a high resolution DEM in a low relief, agrarian landscape, Earth Surf. Proc. Land., 38, 1576–1586, 2013.
Seifritz, W.: Should we store carbon in charcoal?, Int. J. Hydrogen Energ., 18, 405–407, 1993.
Shi, Z. H., Fang, N. F., Wu, F. Z., Wang, L., Yue, B. J., and Wu, G. L.: Soil erosion processes and sediment sorting associated with transport mechanisms on steep slopes, J. Hydrol., 454–455, 123–130, 2012.
Simpson, M. J. and Hatcher, P. G.: Determination of black carbon in natural organic matter by chemical oxidation and solid-state 13C nuclear magnetic resonance spectroscopy, Org. Geochem., 35, 923–935, 2004.
Sohi, S. P., Krull, E., Lopez-Capel, E., and Bol, R.: A review of biochar and its use and function in soil, Adv. Agron., 105, 47–82, 2010.
Sterk, G., Rijksen, M., and Goossens, D.: Dryland Degradation by Wind Erosion and its Control, Ann. Arid Zone, 40, 351–367, 2001.
Stockmann, U., Adams, M. A., Crawford, J. W., Field, D. J., Henakaarchchi, N., Jenkins, M., Minasny, B., McBratney, A. B., de Remy de Courcelles, V., Singh, K., Wheeler, I., Abbott, L., Angers, D. A., Baldock, J., Bird, B., Brookes, P. C., Chenu, C., Jastrow, J. D., Lal, R., Lehmann, J., O'Donnell, A. G., Parton, W. J., Whitehead, D., and Zimmermann, M.: The knowns, known unknowns and unknowns of sequestration of soil organic carbon, Agr. Ecosyst. Environ., 164, 80–99, 2013.
van Boxel, J. H.: Numerical model for the fall speed of raindrops in a rainfall simulator, International Centre for Eremology, Special Report 1998/1, 77–85, 1998.
Wang, C., Walter, M. T., and Parlange, J. Y.: Modeling simple experiments of biochar erosion from soil, J. Hydrol., 499, 140–145, 2013.
Williams, A. T., Rangel-Buitrago, N., Pranzini, E., and Anfuso, G.: The management of coastal erosion, Ocean Coast. Manage., 156, 4–20, 2018.
Zimmermann, M., Leifeld, J., and Fuhrer J.: Quantifying soil organic carbon fractions by infrared spectroscopy, Soil Biol. Biochem., 39, 224–231, 2007.